Amy Sprague
May 12, 2025
A&A Ph.D. student Aditya Deole knows that objects are easier to identify and track if you can see them clearly. He brought this simple insight to A&A's RAIN Lab to improve how we locate and track uncontrolled satellites and debris in the vastness of space.
Aditya Deole's research will facilitate identifying objects in space by approaching at the right angle to take advantage of available sunlight.
Listen to this story:
Aditya Deole, A&A Ph.D. student
Aditya Deole's research addresses a critical challenge in space operations. Machine learning algorithms and neural networks used to locate objects in space are trained on diverse images with varying lighting conditions, but their real-time accuracy fluctuates significantly depending on lighting angles. Deole discovered that these machine learning systems perform most reliably during actual missions when the sun is positioned behind the observing spacecraft, requiring specially designed trajectories to approach objects from optimal viewing angles.
"Sunlight affects image quality," Deole explains. "The best tracking happens when the sun is behind the observer, ensuring the target is well-lit."
This insight led to a sophisticated system that plans spacecraft trajectories not just for fuel efficiency, but for optimal observation angles. The approach has significant implications for space debris removal, satellite servicing and lunar navigation.
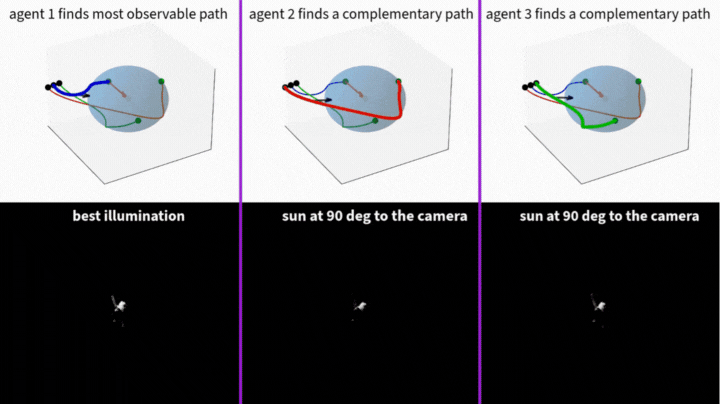
This visualization demonstrates how multiple spacecraft use optimal positioning relative to sunlight to improve space object tracking. The system guides three agent spacecraft to approach a target object from complementary angles, with Agent 1 positioning for optimal sunlight illumination while Agents 2 and 3 provide additional perspectives at 90-degree angles to the sun.
"If we want to track uncontrolled objects like debris, we need some baseline knowledge about them," says Deole. "We train neural networks on simulated images of satellites and debris under various conditions, then design approach paths that maximize recognition accuracy."
A representation of the positioning of the three agent spacecraft in the videos above.
By combining machine learning with traditional trajectory planning, Deole's work addresses a fundamental challenge: reliability. His methodology can measure the uncertainty in neural network predictions, then develop controllers that adjust for these limitations in real time.
"Machine learning has rarely been used for space object training because there are no guarantees that it will work, and space missions demand precision," Deole notes. "Our focus was on understanding when these algorithms might fail."
Professor Mehran Mesbahi, Deole's faculty adviser, highlights the innovative approach in this research. "Aditya's work represents a breakthrough in how spacecraft can see and respond to objects in space," Mesbahi says. "He first created a framework to measure how well machine learning algorithms identify objects under different conditions. Then he designed flight paths that position spacecraft at ideal angles relative to the sun during critical operations. This smart integration creates a robust system for autonomous space missions that experts previously considered too risky."
Deole's methodology extends beyond space applications to autonomous vehicles, robotics and other fields where machine perception guides movement under varying environmental conditions.
"It's about optimizing planning while accounting for neural network biases," Deole explains. "In space, that means sunlight. On Earth, it could be camera angles or sensor placement. The key is understanding when and where a system performs best and planning accordingly."
As space becomes increasingly congested with active satellites and debris, methodologies like Deole's will be essential for safe and efficient operations. His work exemplifies how the UW continues to lead in developing practical solutions to complex aerospace challenges—in this case, just catching some rays from the perfect angle.
Go to the source
Estimation-Aware Trajectory Optimization with Set-Valued Measurement Uncertainties by Aditya Deole and Mehran Mesbahi, January 2025.